Getting Started with Hub
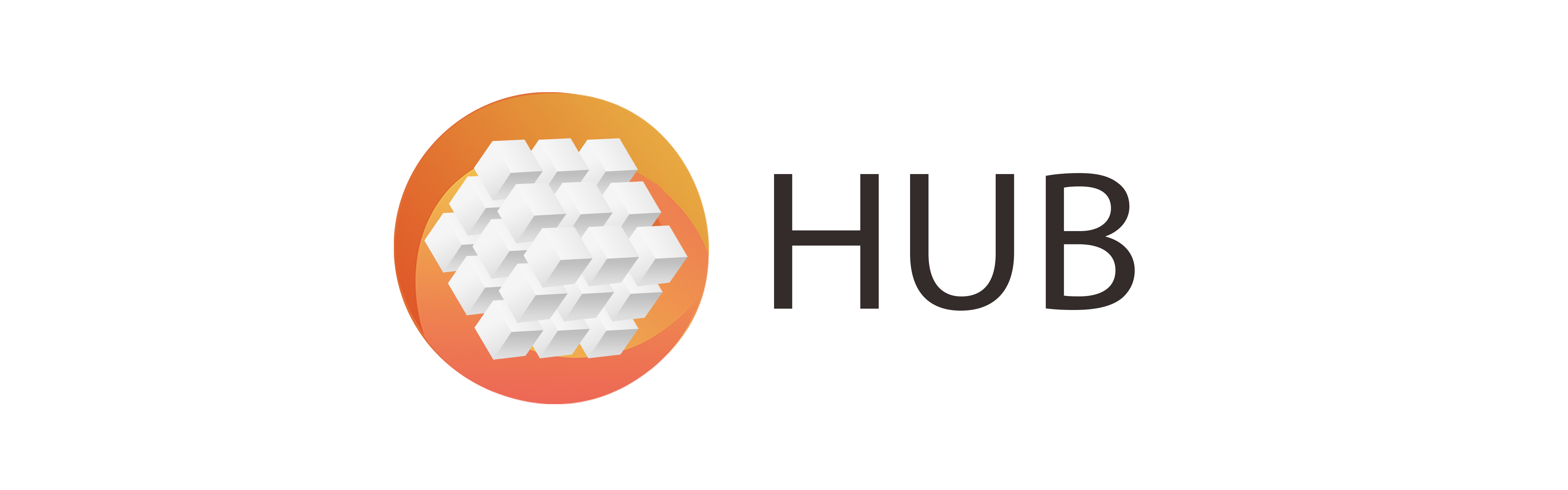
What is Hub?
The fastest way to access and manage datasets for PyTorch and TensorFlow
Hub provides fast access to the state-of-the-art datasets for Deep Learning, enabling data scientists to manage them, build scalable data pipelines and connect to Pytorch and Tensorflow.
Warning
Your project must have the “Internet access” upgrade enabled in order to connect to an online service like Activeloop’s hub from within your project!
Note
This is user-contributed content. Credits go to Activeloop!
Quickstart
Install Hub
pip3 install hub
Register and authenticate to upload datasets to Activeloop store.
activeloop register activeloop login
Alternatively, add username and password as arguments (use on platforms like Kaggle).
activeloop login -u username -p password
Load a dataset
import hub ds = hub.Dataset("activeloop/cifar10_train") print(ds["label", :10].compute()) print(ds["id", 1234].compute()) print(ds["image", 4321].compute()) ds.copy("./data/examples/cifar10_train")
Create a dataset
import numpy as np import hub from hub.schema import ClassLabel, Image my_schema = { "image": Image((28, 28)), "label": ClassLabel(num_classes=10), } url = "./data/examples/quickstart" # write your {username}/{dataset_name} to make it remotely accessible ds = hub.Dataset(url, shape=(1000,), schema=my_schema) for i in range(len(ds)): ds["image", i] = np.ones((28, 28), dtype="uint8") ds["label", i] = 3 print(ds["image", 5].compute()) print(ds["label", 100:110].compute()) ds.flush()
This code creates dataset with 1000 samples in “./data/examples/new_api_intro” folder with overwrite mode. Once the dataset is ready, you may read, write and loop over it.
You can also transfer a dataset from TFDS (as below) and convert it from/to Tensorflow or PyTorch.
import hub import tensorflow as tf out_ds = hub.Dataset.from_tfds('mnist', split='test+train', num=1000) res_ds = out_ds.store("username/mnist") # res_ds is now a usable hub dataset
Data Storage
The first positional argument to declare a Hub dataset is url
.
Hub
If url
parameter has the form of username/dataset
, the dataset
will be stored in our cloud storage.
url = 'username/dataset' ds = hub.Dataset(url, shape=(1000,), schema=my_schema)
You can also create or load a dataset locally or in S3, MinIO, Google Cloud
Storage and Azure. In case you choose other remote storage platforms,
you will need to provide the corresponding credentials as a token
argument during Dataset creation or loading. It can be a filepath to
your credentials or a dict
.
Local storage
To store datasets locally, let the url
parameter be a local path.
url = './datasets/' ds = hub.Dataset(url, shape=(1000,), schema=my_schema)
S3
url = 's3://new_dataset' # your s3 path ds = hub.Dataset(url, shape=(1000,), schema=my_schema, token={"aws_access_key_id": "...", "aws_secret_access_key": "...", ...})
MinIO
url = 's3://new_dataset' # minio also uses *s3://* prefix ds = hub.Dataset(url, shape=(1000,), schema=my_schema, token={"aws_access_key_id": "your_minio_access_key", "aws_secret_access_key": "your_minio_secret_key", "endpoint_url": "your_minio_url:port", ...})
Google Cloud Storage
url = 'gcs://new_dataset' # your google storage (gs://) path ds = hub.Dataset(url, shape=(1000,), schema=my_schema, token="/path/to/credentials")
Azure
url = 'https://activeloop.blob.core.windows.net/activeloop-hub/dataset' # Azure link ds = hub.Dataset(url, shape=(1000,), schema=my_schema, token="/path/to/credentials")
Schema
Schema is a required attribute that describes what a dataset consists of. This is how you can create a simple schema:
from hub.schema import ClassLabel, Image, BBox, Text my_schema = { 'kind': ClassLabel(names=["cows", "horses"]), 'animal': Image(shape=(512, 256, 3)), 'eyes': BBox(), 'description': Text(max_shape=(100,)) }
Shape
Shape is another required attribute of a dataset. It simply specifies
how large a dataset is. The rules associated with shapes are derived
from numpy
.
Dataset Access, Modification and Deletion
In order to access the data from the dataset, you should use
.compute()
on a portion of the dataset: ds['key', :5].compute()
.
You can modify the data to the dataset with a regular assignment operator or by performing more sophisticated transforms.
You can delete your dataset with .delete()
or through Activeloop’s
app on app.activeloop.ai in a dataset
overview tab.
Flush, Commit and Close
Hub Datasets have three methods to push the final changes to the selected storage.
The most fundamental method, .flush()
saves changes from cache to
the dataset final storage and does not invalidate dataset object. It
means that you can continue working on your data and pushing it later
on.
.commit()
saves the changes into a new version of a dataset that you
may go back to later on if you want to.
In rare cases, you may also use .close()
to invalidate the dataset
object after saving the changes.
If you prefer flushing to be taken care for you, wrap your operations on
the dataset with the with
statement in this fashion:
with hub.Dataset(...) as ds: pass
Other information
For more information see Hub documentation .
Join our Slack community for help from Activeloop team and other users as well as dataset management/preprocessing tips and tricks.
For feature requests or bug reports, please open a new GitHub issue.